Introduction / Overview
Welcome to my ePortfolio! I am Franck Fonguila, a graduate student in Statistics at Auburn University. My academic background and research focus are centered on mathematical modeling, risk assessment, and predictive analytics. Through my studies, I have developed a strong foundation in actuarial science and financial decision-making, which I aim to apply in solving real-world financial challenges.
With experience as a Teaching Assistant, I am not only refining my analytical skills but also honing my ability to communicate complex statistical concepts effectively. My goal is to bridge the gap between statistical theory and its practical application in risk analysis and financial forecasting.
🔽 Scroll down to Learn More

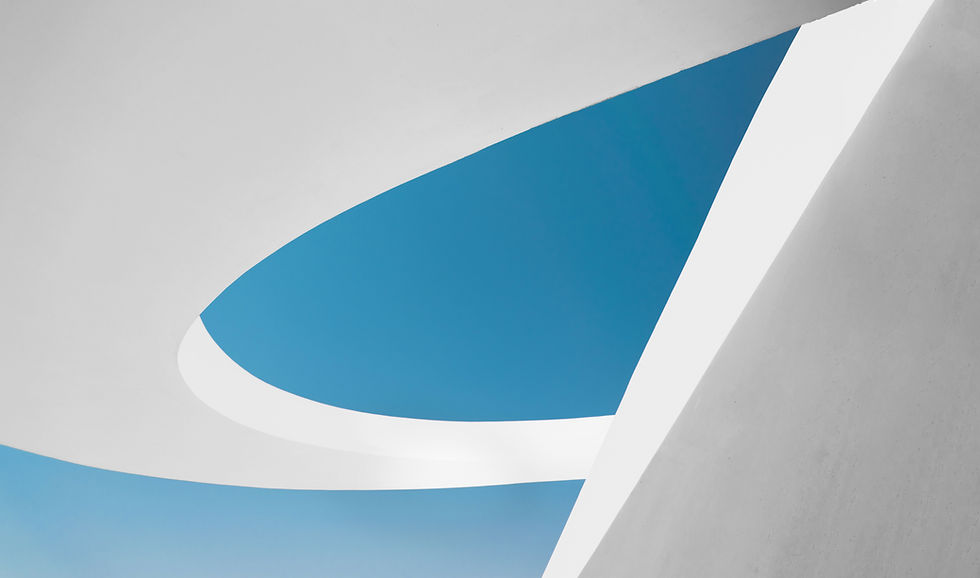
About Me
I have always been fascinated by the power of statistical methodologies in understanding uncertainty and optimizing decision-making. As a graduate student in statistics, I have cultivated a strong analytical mindset and a passion for applying probability, optimization, and data-driven techniques to real-world financial and actuarial challenges.
My role as a Teaching Assistant has provided me with valuable experience in explaining complex statistical concepts to diverse audiences, reinforcing my ability to translate theoretical knowledge into clear, practical insights. Beyond teaching, I am continuously expanding my technical expertise in financial modeling, risk management, and computational statistics, striving to deepen my proficiency in programming and data analysis tools such as Python and R.
I am committed to bridging the gap between statistical theory and its applications in finance, risk assessment, and predictive modeling. Through continuous learning and hands-on research, I aim to develop innovative solutions that support informed financial decision-making in industries such as insurance, banking, and investment analytics.

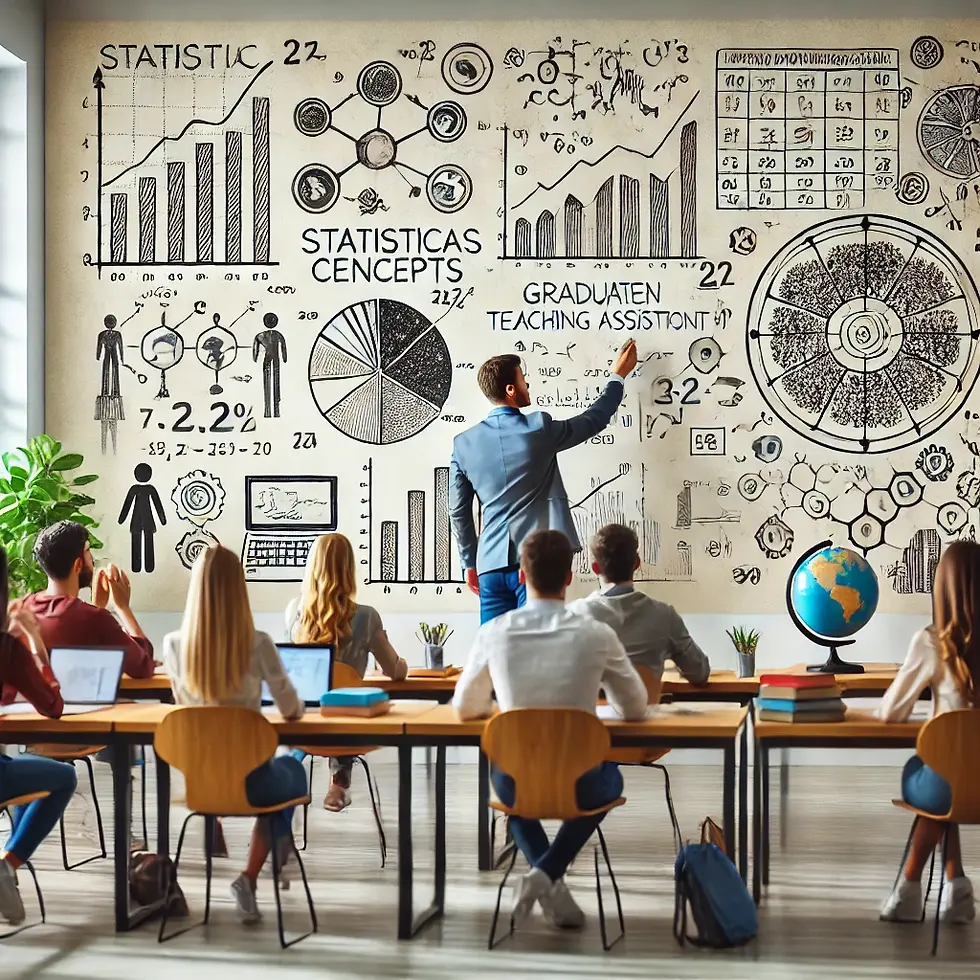
Biographical Statement
Franck Fonguila is a graduate student in statistics at Auburn University in Alabama, USA. He holds a Master’s degree in Mathematics from the University of Douala (Cameroon), where he specialized in economical mathematics. His academic background provides a strong foundation in mathematical modeling, statistical analysis, and optimization techniques.
​
Currently, Franck serves as a teaching assistant in the Mathematics and Statistics departments at Auburn University. In this role, he assists in instructing undergraduate courses, grading assignments, and supporting students in their academic pursuits. This position marks his first experience studying in a U.S. university and teaching in a language that is not his native tongue. To develop strong communication skills, he is currently enrolled in INTL 1830, an English course designed to enhance proficiency in academic and professional communication.
​
Franck’s latest research project was to study how the binomial pricing model works, especially for European options. He explored the basic math behind the model and how it is used in financial markets. Through this work, he gained useful knowledge about financial mathematics and data analysis, improving his problem-solving skills.
​
In addition to his academic and teaching responsibilities, Franck enjoys reading books, watching movies, listening to music, and spending quality time with family and friends. His diverse interests allow him to maintain a well-balanced lifestyle while staying engaged in intellectual and social activities.
​
As a graduate student and aspiring statistician, Franck is going to advance his knowledge in statistical methodologies and their real-world applications. His dedication to research, teaching, and continuous learning positions him as a strong candidate for future academic and professional opportunities in the field of statistics and data analysis.
Research Interest Statement
Calculating the Future: Harnessing Statistics to Manage Risk
I have always been fascinated by how numbers can reveal patterns and guide decisions in the real world. This fascination led me to build a strong foundation in mathematics, a discipline that taught me how to think logically and solve complex problems. Now, as a graduate student in statistics, I am eager to use these skills to tackle practical challenges. One field that particularly captures my interest is actuarial science – the science of using math and statistics to assess and manage risk, especially in insurance and finance. Actuaries use statistical methods to answer questions like, "How likely is an event to happen, and what would the financial consequences be?" For example, they might calculate the probability of natural disasters or accidents and help design insurance policies that protect people when unfortunate events occur. I find this work meaningful because it shows how abstract mathematical ideas can translate into safeguards for communities, businesses, and individuals. While my background in computer science is limited, I am motivated to develop my programming skills as they become increasingly important for analyzing large datasets and improving statistical models. I believe that strong statistical methodologies are the backbone of these efforts – they turn raw data into insights we can trust. Through my studies, I have seen firsthand that even something as theoretical as a probability equation can have real-world impact when applied to decision-making.
​
​My passion for applying mathematics to real-world problems grew even stronger during an undergraduate research project on the binomial pricing model. The binomial pricing model is a step-by-step method for estimating the fair price of financial options (which are contracts that give the right to buy or sell assets under certain conditions). In simpler terms, this model imagines that at each step in time, the price of a stock can either go up or down, like a coin flip. By working through these steps, we can build a "tree" of possible future prices and calculate how much an option should be worth today. In my project, I focused on understanding the theoretical foundations of the model rather than applying it to real-world financial markets. This experience deepened my appreciation for financial mathematics and strengthened my ability to work with complex statistical models. It was thrilling to see a concept from a textbook come alive through computational experiments and simulations. This research also sharpened my ability to communicate complex ideas in simple terms. When I presented my findings, I needed to explain the binomial model to peers who were unfamiliar with it. This meant defining financial jargon in plain language and using everyday analogies. That experience reinforced my belief that no matter how advanced a methodology is, it becomes far more powerful when it is understandable. Moving forward, I am eager to explore more advanced statistical techniques that can help solve pressing problems in risk management. For example, I am interested in improving predictive models for insurance and developing better methods to forecast financial risks. Ultimately, my goal is to become an actuary who not only excels in quantitative analysis but also uses those skills to make a positive difference. Whether it is designing better insurance products or helping businesses make smarter decisions under uncertainty, I want my work to have a meaningful impact. By continuing my graduate research in statistics, I aim to contribute to this field through innovative methods and practical solutions, and always keeping in mind that behind every data point is a real-world story and impact.
​
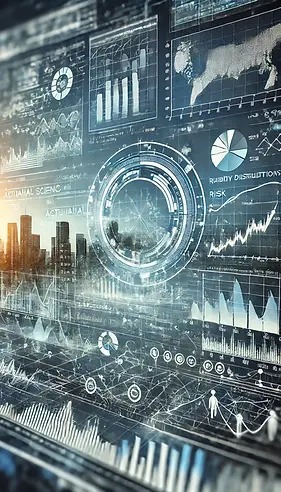
Project Proposal
Title: Improving Regression Models to Handle Outliers and Leverage Points in Actuarial Science
Situation
In actuarial science, statistical models are used to help predict risks, such as the likelihood of accidents, natural disasters, or financial losses. These predictions are important because they help insurance companies and other businesses make decisions about pricing and risk management. However, the models we use sometimes do not work well when there are outliers (extreme data points) or leverage points (extreme values in the predictor variables). For example, if a model is used to predict the cost of insurance but includes data from a rare event, like a massive earthquake, the prediction could be wrong. This is a big problem because it can lead to poor decisions, such as incorrect insurance prices. While there are some methods that try to handle these issues, they are still not perfect, and better solutions are needed.
​
Problem
The main problem with current statistical models is that they are sensitive to outliers and leverage points. For example, the least squares method, which is often used in regression models, can be heavily affected by a single extreme data point. When there is unusual data, such as a very high cost for an insurance claim, the model’s prediction might become unreliable. This is a problem for actuaries who need to make precise risk assessments. Even though there are some methods to reduce the impact of outliers, such as M-estimators and generalized signed-rank estimators, these methods still have some weaknesses, especially when there are extreme values in the predictors (x-space). This means that current methods are not always reliable in real-world situations where data can be messy or unusual.
​
Solution
To solve this problem, the proposal suggests developing a more robust method called a generalized signed-rank estimator. This method is designed to be less sensitive to outliers and leverage points compared to traditional methods like least squares. The idea behind this method is to use a generalized signed-rank norm that minimizes the difference between the observed values and the predicted values, but in a way that gives less weight to extreme data points. This way, the model can focus more on the majority of the data, which is usually more reliable. By applying this method to nonlinear regression models, we can improve the accuracy of the predictions, especially in situations where the data contains extreme values. The project will involve:
1. Reviewing existing methods: Start by looking at the strengths and weaknesses of current regression models, such as least squares, M-estimators, and generalized signed-rank estimators.
​
2. Developing a new estimator: Create a version of the generalized signed-rank estimator that works well with nonlinear models and multidimensional data.
​
3. Testing the new method: Use simulations to test the new estimator on different types of data, especially those with outliers or leverage points.
4. Comparing performance: Compare how well the new estimator works compared to existing methods in real-world scenarios, such as predicting risks in insurance.
Evaluation
The success of this solution will be measured by how well the new estimator handles outliers and leverage points while still giving accurate predictions. The proposal will compare the performance of the new estimator to traditional methods like least squares and M-estimators. To do this, we will use simulation studies that include data with outliers and leverage points to see how each method performs. If the new estimator works better at handling extreme data, it will be considered a successful improvement. We will also evaluate the practical feasibility of the solution. This includes checking how easy it is to implement the new method in real actuarial tasks, such as pricing insurance or managing financial risk. We will also look at the time and computational resources needed to use the new method. Although the method is expected to be efficient, we need to make sure it can be applied in real-life situations where actuaries work with large datasets.
​
Conclusion
This project aims to improve the way we predict risks in actuarial science. By developing a more robust regression method that can handle outliers and leverage points, we can make more accurate predictions. This will help actuaries make better decisions about pricing insurance, assessing financial risks, and managing uncertainties. The proposed generalized signed-rank estimator can reduce the impact of extreme data points while still maintaining the efficiency of the model. If successful, this method could lead to more reliable risk management tools and better decision-making in insurance and finance. The results of this project will provide a more accurate and trustworthy way to analyze data and make predictions, which is crucial for managing financial and insurance risks.
​
References
​
Nguelifack, B. M. (2014). Generalized Signed-Rank Estimator for Certain Complex Regression Models (Doctoral dissertation, Auburn University).
Bindele, G., & Abebe, H. (2012). Generalized Signed-Rank Estimators in Nonlinear Regression. Journal of Statistical Planning and Inference, 142(6), 1662-1676.
​
Teaching & Academic Engagement
As a Graduate Teaching Assistant, I actively contribute to the learning experience of undergraduate students in mathematics and statistics. My responsibilities include:
​
-
Leading tutorial sessions and helping students grasp difficult concepts.
-
Providing guidance on problem-solving approaches in mathematics.
-
Grading assignments and offering constructive feedback to enhance student learning.
This role has strengthened my ability to communicate complex statistical methods in an accessible way, a skill I find invaluable in both academia and industry.
Career Aspirations
My long-term goal is to become an actuary specializing in risk modeling and financial decision-making. I aspire to:
​
-
Use statistical models to assess and mitigate financial risks.
-
Improve predictive analytics techniques to enhance actuarial science.
-
Contribute to data-driven decision-making in finance and insurance.
Skills
Technical Skills
Data Analysis
Statistical Modeling, Predictive Analysis, R Programming
Soft Skills
Analytical Thinking, Problem-Solving, Collaboration
Communication, Time Management
Detail-Oriented, Critical Thinking
Teamwork
Adaptability, Leadership Skills